The FAIR principles
According to the FAIR Guiding Principles for Scientific Data Management, first published in Nature in 2016, research data collected through public funds be accessible to all for a longer period of time. Furthermore, not only people should be able to access and understand the data but also machines. This means that machines should be able to read the data and analyze it with no or limited human involvement (which is one of the key prerequisites for machine learning). This type of artificial intelligence revolves around utilizing the extensive processing capacities of computers to handle or assist with processing data, and in that way meet the increased needs for automation in data analyses of extensive and complicated research data. Since the publication of the FAIR principles, the European Union and numerous international funders and universities have expressed support for the principles and taken them into account in their policy-making.
According to the FAIR principles, "research data should be as open as possible, as closed as necessary". This assumes a degree of flexibility where sensitive data can be placed in restricted access. In this way, research data can be published in restricted or controlled access but still be considered FAIR.
What are the FAIR principles?
The FAIR guiding principles are comprised of 15 principles that describe how scientific data should be organized so it can be more easily accessed, understood, exchanged and reused. These principles are divided into four main components which state that research data should be: Findable, Accessible, Interoperable and Reusable.
Findable
It is important that both people and machines can find the data with as little effort as possible. To this end, research data are assigned a globally unique and persistent identifier, such as a Digital Object Identifier (DOI), which consists of a URL that resolves to a dataset landing page.
Data should also have rich metadata which describes the data and makes sure it is findable through disciplinary local or international discovery portals. The metadata should always be accessible, even if the data itself is unavailable.
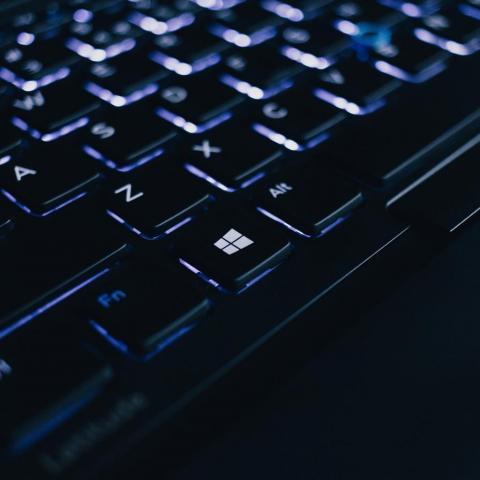
Accessible
Data and metadata should be assigned a clear user license that is understandable to both people and machines. This may include making the data open using a standardized protocol. However, the data does not necessarily have to be open (such as sensitive data). Examples of sensitive data include privacy concerns or national security. When it is not able to be open, there should be clarity and transparency around the conditions governing access and reuse.
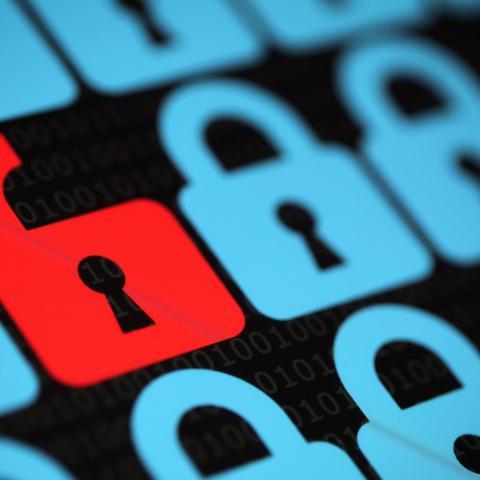
Interoperable
Data and metadata should use community accepted languages, formats and vocabularies. Metadata should reference and describe relationships to other data, metadata and information through using identifiers.
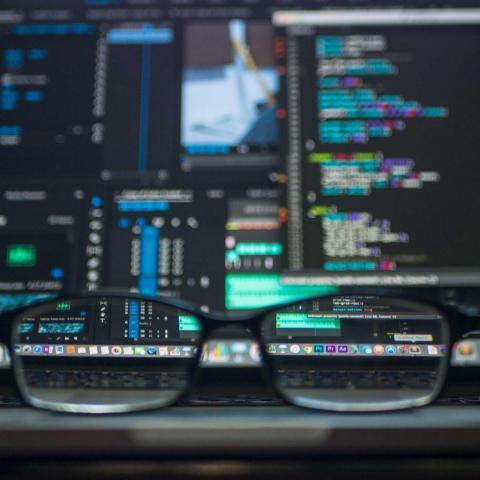
Reusable
Reusable data should maintain its initial richness. For example, it should not be diminished for the purpose of explaining the findings in one particular publication. It needs a clear machine-readable licence and provenance information on how the data was formed. It should also have discipline-specific data and metadata standards to give it rich contextual information that will allow reuse.
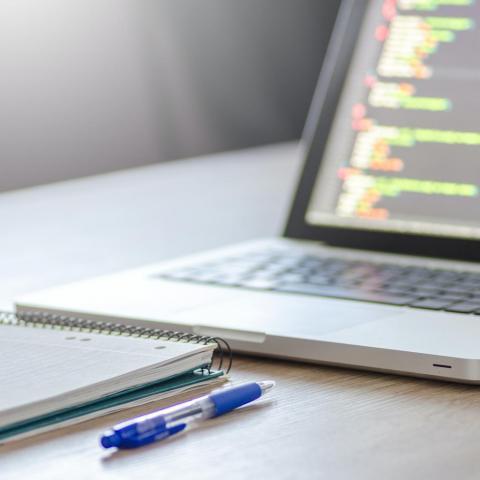